AI & Data Governance
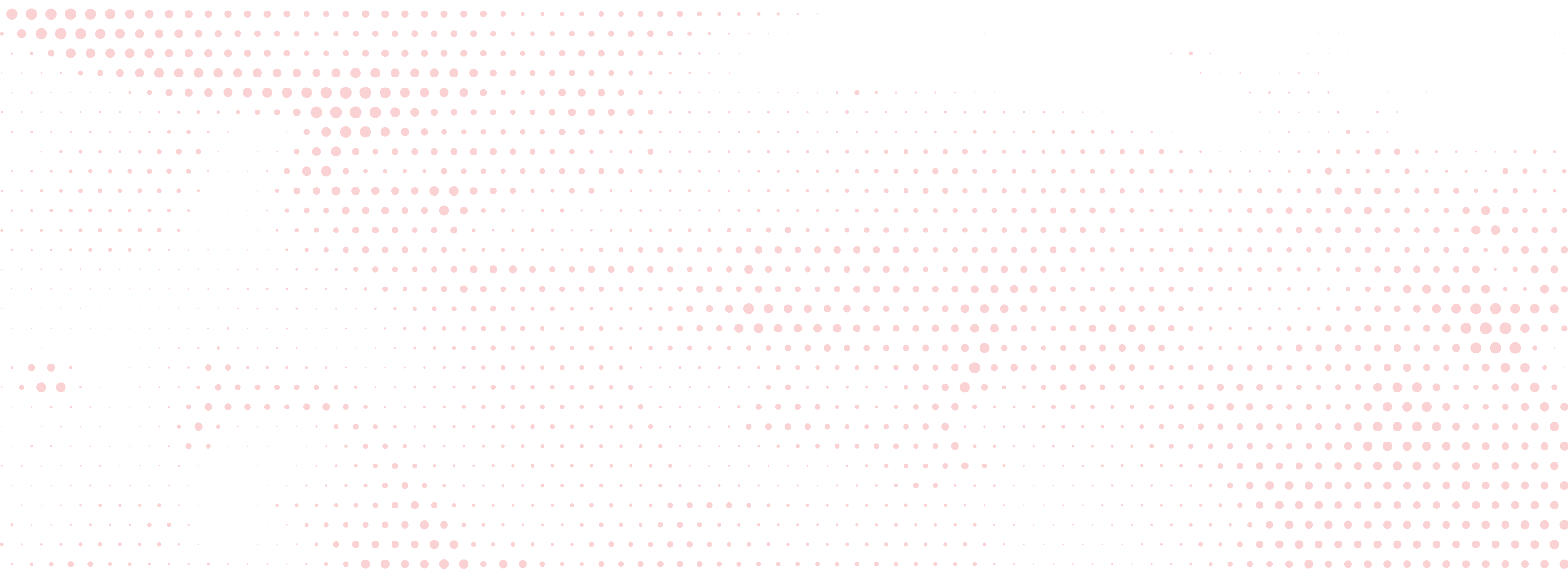
This blog discusses how the rise of AI is forcing organizations to finally take data governance seriously since AI's ability to learn and make autonomous decisions means poor data quality and management are riskier than ever before.
Improving Data Governance and Management – Why Now Is the Time to Get It Right
Data governance has been an essential, if sometimes underappreciated, area of enterprise management for decades. Despite countless efforts, major gaps still exist—data quality remains inconsistent, lineage tracking is often incomplete, and governance policies are frequently inadequate or not fully implemented. With AI, however, this can't be business as usual. Unlike traditional systems, AI doesn’t just process data—it learns from it, adapts based on it, and even makes decisions that impact people, industries, and lives. This makes data quality, accuracy, and transparency no longer just desirable but essential.
Why AI Is a Catalyst to Improve Data Governance
AI has surfaced long-standing cracks in enterprise data management, making them impossible to ignore. The risks are real: a poor-quality dataset can lead to untrustworthy, unethical, and even dangerous AI decisions. The stakes are higher than ever because some AI models make autonomous decisions; they can be “agentic” in ways that traditional systems are not. As a result, poor data governance risks have skyrocketed, putting pressure on organizations to finally address these critical issues.
Without high-quality data, AI simply will not work as intended. Models trained on poor, incomplete, or inaccurate data produce unreliable and skewed results, eroding trust. Imagine relying on an AI model to recommend medical treatments, but the data it learned from lacks diversity, doesn’t reflect recent medical advancements, or contains errors. In scenarios like this, poor data governance becomes a direct threat not only to business success but also to individual well-being and regulatory compliance.
The AI Opportunity for Real Data Governance
AI can, and should, be the motivation enterprises need to address data governance comprehensively. Here’s why:
- Elevated Standards for Data Quality: AI is unforgiving when it comes to data quality. Unlike traditional applications that can function with certain allowances for inconsistency, AI thrives only on clean, accurate, and representative data. Organizations are now incentivized to ensure data quality because AI models reveal flaws quickly, and poor-quality data undermines both model performance and compliance.
- Data Lineage for Transparency and Compliance: AI, especially in regulated industries, is subject to increasing scrutiny around decision transparency. Data lineage, which tracks the origins and transformations of data, is no longer just a “best practice” but a regulatory expectation. Companies need clear, accurate lineage to explain AI decisions, making it a core pillar of data governance.
- Agentic AI and Trustworthiness: Trustworthy AI cannot exist without robust data governance. Agentic AI systems that make critical decisions must have reliable, well-documented data foundations to avoid bias, maintain transparency, and ensure fair treatment of individuals. This shift pushes companies to adopt governance practices that mitigate the risks of bias, misuse, or data-related legal issues.
- Data Privacy and Security: With personal data fueling many AI models, regulatory requirements around privacy and security have grown stringent. AI compliance with GDPR, CCPA, and similar regulations hinges on robust data governance. These regulations mandate clear accountability, consent management, and data minimization—all achievable only through a mature governance framework.
How AI Itself Can Help Transform Data Governance
Interestingly, AI also holds the potential to enhance data governance practices, helping enterprises overcome challenges in managing data spread across multiple systems and clouds. By integrating an ontological approach—using AI to create an organized, contextual understanding of data across various systems—companies can more effectively manage and govern dispersed data.
- Ontological Data Mapping Across Systems and Clouds: AI-powered ontologies allow organizations to create interconnected maps of data residing in different systems and clouds, providing a holistic view. This approach enables organizations to manage data relationships, track its lineage across complex ecosystems, and ensure consistency in governance practices across disparate environments.
- Automating Data Quality and Cleansing: AI-driven tools can detect anomalies, correct inconsistencies, and flag potential errors, automating a significant portion of data quality management. With AI, organizations can move from reactive data quality measures to proactive, continuous improvement.
- Intelligent Access Control and Privacy Management: AI can monitor data access patterns, detect unusual behaviors, and enforce fine-grained, dynamic access controls. With this capability, organizations can better safeguard sensitive data, ensuring that access policies adapt to real-time conditions and enhance overall compliance.
- Bias Detection and Mitigation at Scale: AI can detect biases in data before they become ingrained in models, allowing organizations to make adjustments before model deployment. Furthermore, by continuously monitoring deployed AI systems, AI-based tools can highlight potential shifts in model behavior, ensuring ongoing fairness and compliance.
Making the Case: A Data Governance Roadmap for AI
To realize the full value of AI and protect against its risks, organizations need a comprehensive data governance roadmap that includes:
- Policy Alignment and Role Designation: Establish policies that align with AI-specific needs, assigning clear roles such as data stewards and data owners.
- Data Quality and Diversity Standards: Set explicit quality standards and ensure datasets are representative, balanced, and free from inherent biases.
- Integrated Data Lineage Tracking: Use AI to manage data lineage across systems, ensuring traceability from data sources to AI decisions.
- Real-Time Monitoring and Continuous Feedback: Implement AI-driven, continuous validation processes, coupled with real-time feedback loops to ensure data maintains high standards.
Conclusion: Transforming Data Governance to Deliver Value with AI
AI has made data governance both an urgent priority and an opportunity for transformation. By addressing data governance through the lens of AI, organizations can build resilient, compliant, and trustworthy AI systems. This is the era for organizations to take data governance seriously—to go beyond checklists and build robust frameworks that turn data into a true asset, supporting not only AI initiatives but the organization’s broader digital and ethical goals.
AI is here to stay, and with it, the demand for rigorous data governance has arrived. Those who seize this moment will not only be compliant and competitive; they will be leaders in the next generation of responsible, trustworthy AI.
In the next blog, we will provide practical, actionable guidance on preparing your data governance program and data assets for AI-driven transformation.
Featured in: AI / Artificial Intelligence