AI Governance Organizations Must Evolve for a New Era
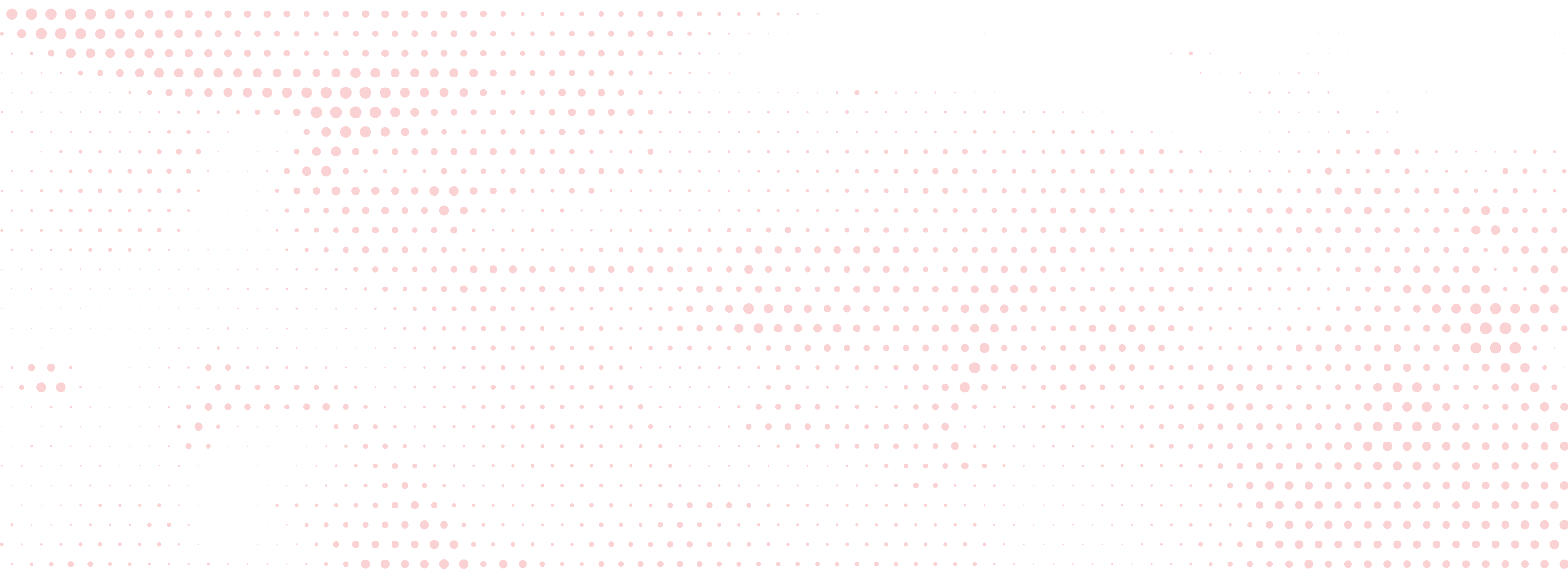
This blog outlines how organizations must adopt dynamic, AI-powered governance frameworks to effectively manage artificial intelligence systems while fostering innovation and maintaining ethical standards in a rapidly evolving technological landscape.
The Stakes Couldn’t Be Higher
What happens when the technologies we build to serve us begin to outpace our ability to control them?
Artificial intelligence (AI) isn’t just another technological advancement—it’s a transformative force reshaping industries, redefining competition, and challenging traditional approaches to governance. Outdated governance frameworks, designed for static and predictable systems, are ill-equipped to handle AI’s dynamic, evolving nature.
AI introduces unique complexities and risks that require organizations to think differently. Inadequate governance of AI can result in reputational damage, loss of business, regulatory penalties, and the erosion of public trust. AI can operate autonomously, often learning, making decisions, and acting alone. This capability is both a strength and a risk, introducing unprecedented challenges such as unintended bias, ethical misuse, and regulatory complexities.
But governing AI isn’t just about compliance; it’s about fostering trust, resilience, and innovation in an era of constant change. To rise to this challenge, organizations must embrace new paradigms for governance, with structures as adaptive, intelligent, and dynamic as the AI systems they aim to control.
("AI governance refers to the framework of systems, policies, and procedures an organization implements to manage and oversee AI initiatives." OnStrategy)
Why Traditional Models Fall Short
AI governance demands something radically different. It’s no longer enough to assign static roles or impose rigid processes. Consider the unique features of AI:
- Autonomy: AI systems make decisions independently, often without direct human intervention.
- Complexity: Advanced models like neural networks operate as “black boxes,” with the basis for outputs being difficult to interpret even for experts.
- Rapid Evolution: AI technologies and regulations are changing so quickly that static governance frameworks could become obsolete almost overnight.
Without an agile, forward-thinking governance model, organizations risk reputational damage, regulatory penalties, and loss of stakeholder trust. Conversely, getting it right unlocks AI's full potential while safeguarding against its risks.
Dynamic Governance: A New Paradigm
To govern AI effectively, leaders should move beyond static hierarchies and structures and adopt agile, collaborative organizational frameworks that can adapt to new challenges.
Governance by Design
Just as “privacy by design” transformed data protection, organizations must embed governance into every stage of the AI lifecycle:
- During model development, conduct governance checkpoints such as automated fairness audits or bias testing.
- At deployment, use real-time monitoring to flag and address potential risks before they escalate.
- Throughout operations, integrate AI-driven tools to continuously assess compliance and ethical alignment.
Governance Sprints
Borrowing from Agile methodology, organizations should implement governance sprints - short, focused cycles where cross-functional teams assess and address emerging AI risks. These sprints allow organizations to respond quickly to new regulations, technologies, or challenges.
AI Risk Labs
Establish collaborative hubs that bring together ethicists, data scientists, business leaders, and compliance experts. These “AI Risk Labs” can co-design governance solutions tailored to specific AI applications, fostering innovation while maintaining ethical and regulatory alignment.
Bridging Innovation and Organizational Structure
To implement dynamic governance strategies like Governance by Design or AI Risk Labs, organizations need well-defined roles and responsibilities. Each role must be designed to support agility, collaboration, and continuous oversight. Below is a framework for structuring AI governance to align with these principles.
Flexibility: A Core Strategic Advantage
Governing AI in a constantly changing landscape requires flexibility. Effective governance must resemble a living organism, able to adapt, grow, and respond to the rapidly changing environment as AI applications expand.
For example:
- Adaptive Policies: Rather than creating static policies, design modular frameworks that can evolve as AI capabilities and regulations change.
- Scalable Structures: Build governance models that can scale from small pilot projects to enterprise-wide AI deployments.
Achieving flexibility requires governance roles that can support dynamic collaboration, as outlined in the sections below.
Using AI to Govern AI: A Paradigm Shift
Perhaps the most transformative idea in AI governance is leveraging AI itself to enhance oversight. This “AI governing AI” approach uses machine learning and automation to monitor, evaluate, and refine AI systems in ways that manual processes cannot match. "AI-driven tools can monitor systems for anomalies, detect biases in real time, and predict risks before they escalate." Reuters
Use Cases:
- Real-Time Risk Mitigation: AI tools can detect and halt high-risk decisions autonomously, reducing human error and response times.
- Bias Evolution Monitoring: Advanced algorithms can track and address bias as models evolve or as datasets shift over time.
- Continuous Compliance: AI-driven systems can ensure adherence to regulatory requirements dynamically, across jurisdictions.
In this paradigm, governance isn’t just a safeguard—it’s a driver of trust, efficiency, and competitive advantage. The organizations that embrace this approach will set the standard for responsible AI innovation.
Organizational Structures and Responsibilities for AI Governance
To operationalize these innovative approaches, organizations must define and align roles to ensure accountability and adaptability. Below are key elements of an effective AI governance structure:
1. Executive Leadership and Oversight
- Chief AI Officer (CAIO) or AI Governance Committee: Oversees AI strategy, ethical considerations, and risk management, ensuring alignment with broader organizational goals. "As generative AI becomes increasingly central to business strategies, many companies have established the role of Chief AI Officer (CAIO)." Financial Times
- Board of Directors: Provides top-level oversight, reviewing AI risks, compliance status, and strategic alignment.
2. AI Risk Management Office
- AI Risk Manager/Officer: Identifies, assesses, and mitigates AI risks, collaborating with compliance and legal teams.
- AI Compliance Team: Ensures adherence to regulations, standards, and ethical guidelines.
- Ethics and Responsibility Office: Develops frameworks for transparency, fairness, and responsible AI use.
3. Data and Technology Leadership
- Chief Data Officer (CDO): Manages data quality and infrastructure, essential for reliable AI systems.
- Chief Information Security Officer (CISO): Oversees AI-related security risks and protects against cyber threats.
4. Legal and Regulatory Compliance
- General Counsel: Ensures compliance with data privacy laws, AI-specific guidelines, and ethical standards.
- Privacy Officer: Focuses on managing privacy risks related to AI.
5. Cross-Functional Teams
- Data Science and Engineering Teams: Build AI models with fairness and bias mitigation in mind.
- Model Validation Team: Independently stress-tests AI models for robustness and compliance.
- AI Product Management: Aligns AI products with governance goals, translating ethical considerations into actionable product features.
6. Independent Auditing and Assurance
- Internal Audit Function: Conducts regular audits of AI systems for compliance and performance.
- External AI Auditors/Consultants: Provides independent evaluations to validate compliance and ethical standards.
7. Training and Culture
- AI Ethics and Compliance Training: Educates employees on AI risks and governance principles.
- AI Champions or Ambassadors: Promote responsible AI use across departments.
Expanding Governance for Emerging AI Frontiers
While today’s governance challenges are formidable, organizations must also prepare for future paradigms:
- AI Generating AI: How will organizations govern systems that autonomously create new models?
- Edge AI: Governance must adapt to decentralized systems making real-time decisions.
- Foundation Models: Address risks related to massive pre-trained models like GPT or BERT.
By anticipating these challenges now, organizations can position themselves as leaders in the next wave of AI-driven transformation.
Closing the Gap: A Call to Action
AI governance isn’t a one-time initiative. It’s a continuous journey that requires commitment, innovation, and collaboration.
Organizations must:
- Evaluate their current governance structures for flexibility, scalability, and adaptability.
- Invest in AI-driven tools to enhance oversight and compliance.
- Collaborate across industries and with regulators to co-create governance standards.
The future of AI is here. The question is: Will your governance frameworks be ready to meet it? Let’s build governance systems that don’t just manage AI but elevate it - unlocking its full potential while safeguarding trust, integrity, and innovation.
Featured in: AI / Artificial Intelligence