How to Blend AI Governance with Existing GRC Programs
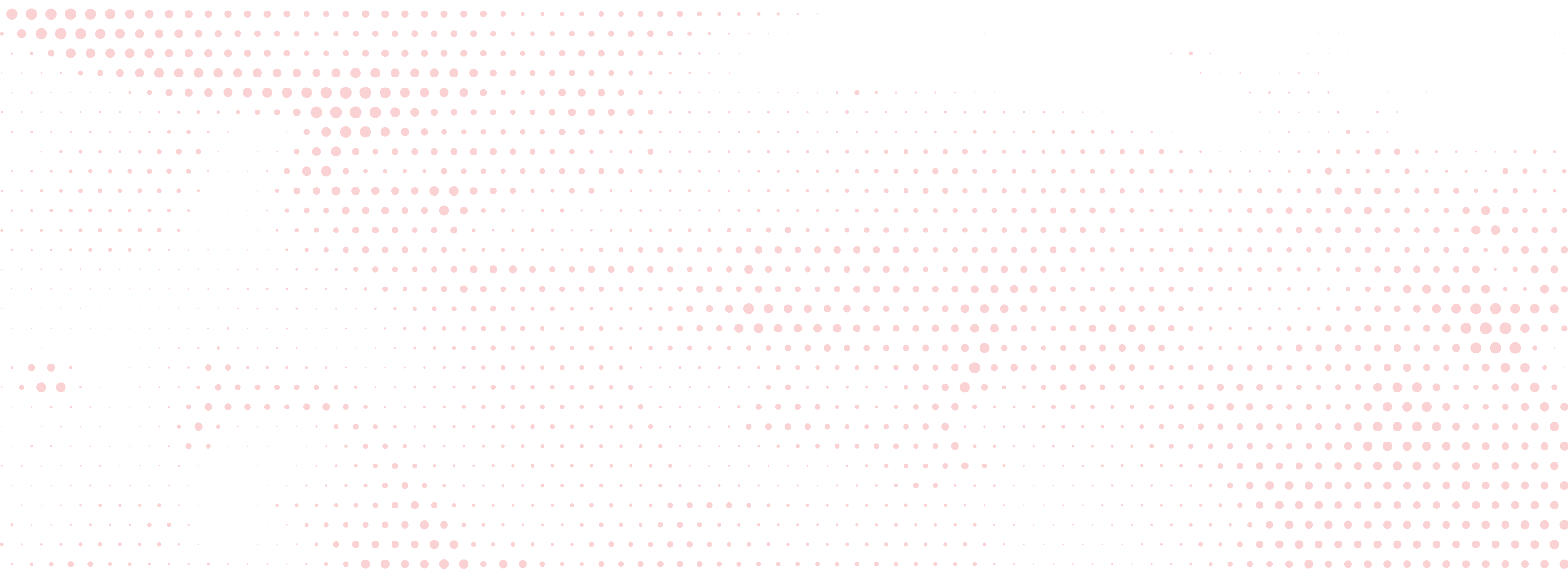
The article provides a comprehensive, step-by-step guide for organizations to integrate AI governance into their existing Governance, Risk, and Compliance (GRC) programs by leveraging current frameworks while addressing AI-specific challenges like model drift, bias, and transparency.
Blending AI Governance with Existing GRC Programs: How to Make It Work Without Completely Reinventing the Wheel
What practical steps can companies take to integrate new AI governance with existing GRC programs? What is new and different? What parts of existing GRC programs can be leveraged to govern and manage the risks of AI? As AI continues to shape the business landscape, these are common and to-be-expected questions.
Integrating AI governance with existing GRC (Governance, Risk, and Compliance) programs is no longer just a good idea—it's essential. But here’s the thing: AI brings some unique quirks to the table, which means it can't be managed in the same way as your standard IT system. So, how can companies navigate these new waters while building on the solid foundation they already have? Let’s break it down into practical steps.
Step 1: Expand What You’re Already Doing to Include AI Risks
Leverage What You Have: Most companies already have some version of a GRC framework. This means you're familiar with managing financial, operational, and regulatory risks. Good news—you don’t have to scrap everything and start over! Your existing risk and compliance processes can be adapted to handle AI. Just widen the lens a bit to account for things like AI bias, explainability, and transparency.
What’s New? AI isn’t your typical static technology. It learns and evolves, which means the risks evolve too. Bias and fairness, for example, aren't just ethical concerns—they're central risks that need to be managed in real time. AI models can drift, meaning they may behave differently over time as data changes. So, it's not enough to set up rules and walk away. AI governance demands constant vigilance.
Step 2: Create a Cross-Functional AI Governance Committee
Use What You’ve Got: Most organizations have committees for risk management, compliance, or IT governance. Great! Just make sure you expand these teams to include AI experts. Bring in your data scientists, IT leads, legal, and maybe even an ethics officer.
Why Is This Different? AI is a team sport, and it’s a sport with technical and non-technical players. You’ll need people who understand the nuts and bolts of machine learning sitting alongside those who are laser-focused on legal and ethical implications. This ensures everyone speaks the same language (or at least understands the translations) when it comes to monitoring AI behavior, bias, and performance.
Step 3: Update Risk Frameworks for AI-Specific Risks
Lean on Existing Practices: You've probably already got a risk assessment process in place for cyber threats, regulatory compliance, and operational failures. That process works as a starting point for AI, but you’ll need to throw some AI-specific risks into the mix.
What’s New? AI risks come with their own baggage. Think about things like model drift (AI systems degrading over time), algorithmic bias (AI treating some groups unfairly), and adversarial attacks (bad actors intentionally messing with your AI’s inputs). You’ll need new categories and metrics to measure these, but the good news is you can add them into the risk management programs you already have.
Step 4: Align AI Governance with Regulatory Compliance
Start with What You Have: If you’re already compliant with data protection regulations like GDPR or HIPAA, you’re part way there. Just add AI into the mix. Look into upcoming AI-specific regulations like the EU AI Act, which is likely knocking on your door.
What’s Different? AI governance introduces new layers to compliance. It’s no longer just about protecting data—it’s about explaining what your AI is doing with that data. Transparency and explainability are buzzwords you’ll need to get familiar with, because they’ll be baked into future regulations. Also, keep an eye on fairness and accountability—areas where AI-specific rules are evolving fast.
Step 5: Build in Continuous Monitoring and AI Audits
Use Your Internal Auditing Function: Your company likely has internal auditing processes in place for financial, operational, or IT compliance. Good news: you don’t have to start from scratch here, either. Just build out your audit process to include AI systems. You will likely need some new skills and resources to address AI in all its forms and circumstances.
What’s New? AI systems need a bit more TLC than traditional audits provide. Continuous monitoring is key, especially when it comes to detecting bias, monitoring performance, and keeping an eye on model drift. Unlike regular IT systems, AI is always learning, so audits can't just happen once a year—they need to be ongoing. This requires new technology and new tools.
Step 6: Integrate AI-Specific Controls
Stick with What Works: You’ve got internal controls for things like IT security and financial reporting. These frameworks can be expanded to cover AI models too.
What Needs Changing? AI-specific controls are a must. That means building in mechanisms to detect and mitigate bias, ensuring AI decisions can be explained to humans, and setting up version controls to track model updates. This isn’t just about ticking boxes; these controls will help build trust with customers and regulators alike.
Step 7: Beef Up Data Governance for AI
Leverage Existing Data Governance: Most organizations already have some level of data governance in place, aimed at ensuring data quality and privacy. The key here is to extend that to the data you’re feeding into your AI systems. At most companies, the bar will be raised and the effectiveness of data governance programs will need to be challenged and improvements made.
What’s Different? AI systems are only as good as the data they’re trained on. This means data governance must take on new tasks—like making sure training data is free of bias, ensuring privacy is maintained during data collection, and keeping tabs on data provenance so you can trace the lineage of every piece of data your AI touches. Expect to need to enhance or add capabilities, systems and processes.
Step 8: Train, Train, Train (and Then Train Some More)
Build on Existing Training Programs: Most companies already run training programs on compliance, cybersecurity, and data privacy. Now you need to add AI governance training to an already demanding workload.
What’s New? Training for AI governance will need to include lessons on AI ethics, how to detect bias, and explainability. Non-technical teams, like legal and HR, should also be trained to understand AI’s impact on their areas, so they can evaluate it properly.
Step 9: Foster Strong Collaboration Between AI and GRC Teams
Use Your Cross-Functional Frameworks: If your company already encourages collaboration among IT, legal, and compliance, you’re in good shape. Just widen the circle to include AI specialists and other relevant stakeholder groups.
What’s Different? AI risks are complex and technical, so collaboration among GRC and AI professionals isn’t just nice to have—it’s essential. AI specialists need to work hand-in-hand with legal, compliance, and risk management to make sure AI doesn’t become a rogue actor in your risk landscape.
Conclusion: AI Governance Isn’t a Reinvention—It’s an Evolution
At the end of the day, integrating AI governance into your existing GRC programs doesn’t require a complete overhaul. But it does demand a significant evolution. The key is recognizing where your current frameworks can be extended and where AI’s unique challenges need new solutions. Yes, it’s more complex, but it’s also an opportunity to build smarter, more resilient governance processes. Done right, AI governance won’t just keep you compliant—it will make your entire GRC framework stronger and more adaptable for the future.
Featured in: AI / Artificial Intelligence