Confronting the AI Bias Monster
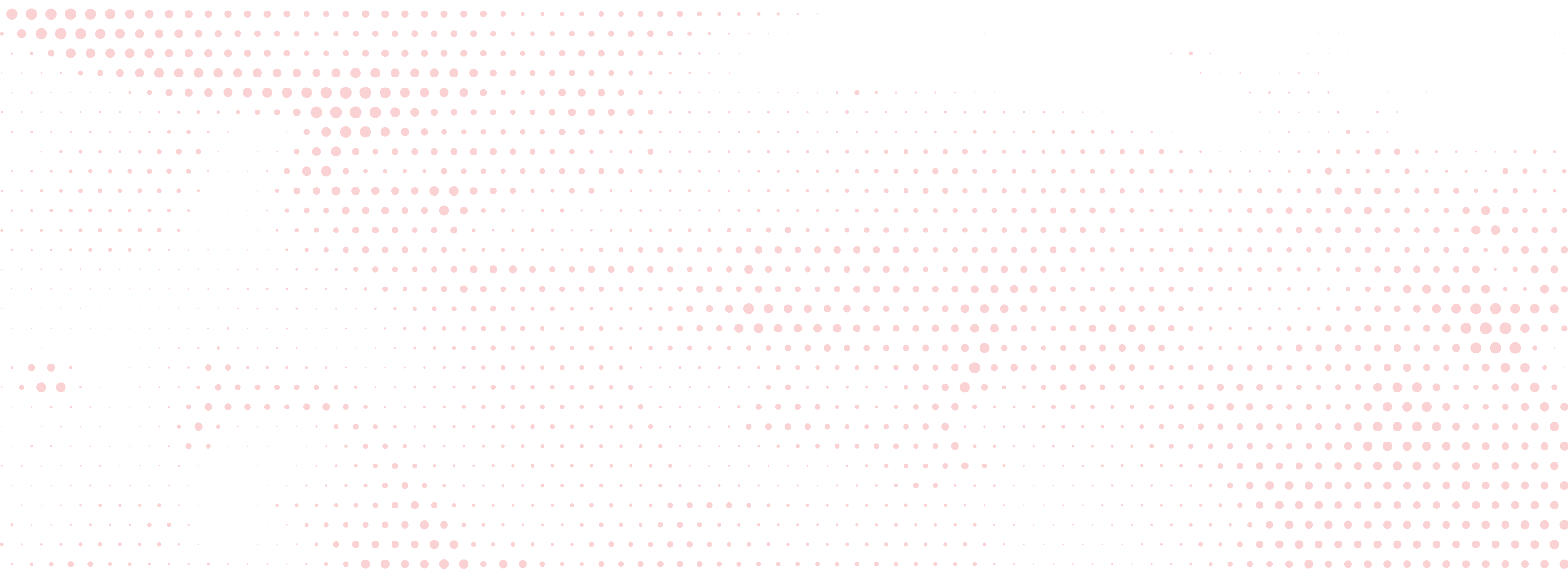
This blog discusses the critical importance of identifying, understanding, and mitigating bias in artificial intelligence systems, emphasizing that AI bias is not just a technical challenge but a complex governance issue with far-reaching societal and business implications that requires proactive strategies, continuous monitoring, and a holistic approach to ensure fairness, transparency, and accountability.
A Wake-Up Call for CEOs: Hidden Bias in AI Could Destroy Your Brand
Bias in AI isn't just an ethical dilemma. It could be a ticking time bomb for your enterprise. If your AI applications reinforce unfair practices or deliver discriminatory results, you're risking regulatory fines, inviting public backlash, and potentially losing market trust that could take years to rebuild.
The real question isn’t whether your AI has any bias - it’s whether you’ve done enough to understand it before you’re in the headlines. In today’s digital world, some algorithmic decisions have the potential to become a front-page scandal. CEOs who fail to manage this risk are gambling with their brand's reputation and their company’s future.
Bias Isn't Always Wrong, But Ignoring Its Implications Is
AI systems learn from data, and that data often mirrors the world as it was, not as we want it to be. Bias in historical data may not mean the data is incorrect—it may, in fact, provide an accurate snapshot of past behaviors, priorities, and societal norms. For example:
- Credit scoring data might reveal systemic underrepresentation of certain groups in loan approvals—not because they were less qualified, but because of structural inequities in lending practices.
- Hiring data might reflect a legacy of workplace discrimination that excluded certain demographics from certain roles or industries.
These biases tell a story - a story of the past. The challenge is that deploying AI without interrogating these biases risks perpetuating outdated norms in a world that increasingly demands fairness, inclusivity, equity, and accountability.
This leads to a critical question: Is your organization prepared to navigate the gap between historical truths and contemporary values? More importantly, can you articulate the implications of that gap to your stakeholders including employees, customers, regulators, and the public?
Understanding the multifaceted nature of bias begins with a clear definition and the contexts in which it arises.
What is AI Bias?
AI bias refers to systematic and unfair discrimination that arises in AI outcomes due to skewed data, flawed algorithms, or human oversight. At its core, AI bias undermines the principles of fairness, accuracy, and reliability that AI systems aim to uphold.
Bias is not a mere technical flaw but a critical governance issue. Left unaddressed, bias erodes trust, diminishes fairness, and jeopardizes compliance with emerging regulations. As regulators, businesses, and society at large scrutinize AI systems, it has become imperative for organizations to make bias mitigation a cornerstone of their AI governance programs.
(For further reading about AI Bias, check out these two papers:
“Fairness and Bias in Artificial Intelligence: A Brief Survey of Sources, Impacts, and Mitigation Strategies” - https://www.mdpi.com/2615402” and
"Identifying and Addressing Bias in Artificial Intelligence” - https://jamanetwork.com/journals/jamanetworkopen/fullarticle/2822033.)
The Multi-Dimensional Nature of AI Bias
Data Bias
Bias often originates in the data used to train AI models. Historical imbalances, underrepresentation, or outright errors in datasets can skew outcomes.
Example: AI models for hiring may underrepresent women or minority groups due to past biases in recruitment data.
Biased data isn’t inherently inaccurate. Historical data often reflects the reality of past practices and decisions, even if those practices were unfair or inconsistent with modern values. This presents a dual-edged challenge and opportunity for organizations leveraging AI.
Algorithmic Bias
Flaws in model design, such as weighting factors or feature selection, can reinforce stereotypes or create unintended disparities.
Example: Predictive policing systems disproportionately target certain communities due to biased weighting of historical crime data.
User and Operational Bias
Even well-designed systems can become biased due to user behavior or contextual factors during deployment.Example: Feedback loops in recommendation systems can perpetuate inequities by amplifying popular but biased content.
The Role of Regulation and Standards
Emerging Laws
Regulations such as the EU AI Act and the U.S. AI Bill of Rights establish stringent requirements around bias detection and mitigation.
Ethical Guidelines
Industry frameworks, including those by IEEE, OECD, and NIST, offer principles for fairness and transparency.
Proactive Compliance
Align organizational practices with regulatory standards to avoid reactive risk management.
Real-World Consequences of Bias
Societal Impact
Biased AI outcomes in hiring, credit scoring, healthcare, and law enforcement have led to real-world harm, including discrimination and loss of opportunities.
Example: An AI-based healthcare system prioritizing treatment for certain groups over others due to skewed training data.
Business Risks
Organizations face reputational damage, customer distrust, regulatory penalties, and legal liabilities due to unchecked bias.
Example: Regulatory fines levied against companies for deploying discriminatory AI systems.
Economic Inefficiencies
Bias reduces the ability of businesses to effectively serve diverse markets, limiting innovation and market reach.
Governance Strategies to Address Bias
Accountability Frameworks
Assign clear roles and responsibilities for identifying, mitigating, and overseeing bias. Ensure board-level oversight of AI governance programs, with special attention to bias considerations.
Data Governance for Bias Prevention
- Use diverse and representative datasets.
- Audit data for completeness, accuracy, and fairness.
- Include ontological approaches to harmonize definitions and relationships in data.
Bias Detection in Development
- Deploy tools to quantify bias in model training.
- Utilize fairness metrics such as demographic parity or equalized odds.
- Include cross-disciplinary teams to evaluate ethical implications.
Monitoring Post-Deployment Bias
- Continuously evaluate AI outputs in real-world settings.
- Establish mechanisms for end-user feedback and issue reporting.
Explainable and Transparent AI
Ensure stakeholders can understand and trust AI outputs and decisions, especially in high-stakes applications like healthcare or finance.
The Role of AI in Addressing Bias
Bias Mitigation Tools
Leverage AI itself to identify and address biases in datasets and algorithms.
Ontological Approaches
Employ shared semantic layers to ensure fairness and consistency across diverse systems and datasets.
Synthetic Data
Generate synthetic data to supplement underrepresented groups, reducing inherent biases in training datasets.
Moving Beyond Technical Fixes
Cultural Change
Promote awareness and inclusion within development and deployment teams.
Interdisciplinary Collaboration
Involve ethicists, domain experts, and sociologists in AI lifecycle governance.
Stakeholder Engagement
Engage impacted communities to ensure AI systems align with their needs and values.
Generative AI: A Mirror of Society's Biases
Generative AI models, such as large language models (LLMs) and image generation systems, are trained on vast datasets scraped from the internet and other sources. While this approach enables these models to generate realistic and creative outputs, it also exposes them to the biases embedded in the underlying data. For example, AI-generating job descriptions might perpetuate gender stereotypes by associating technical roles with men and caregiving roles with women. Similarly, image-generation tools trained on biased datasets may create visuals that reinforce racial or cultural stereotypes.
The societal impacts of these biases are profound. Generative AI outputs influence public perceptions, marketing content, and decision-making in industries ranging from advertising to education. When unchecked, these biases can amplify harmful stereotypes, marginalize underrepresented groups, and erode trust in AI technologies.
Mitigating Bias in Generative AI
Addressing bias in generative AI requires proactive strategies:
Data Curation and Preprocessing: Training datasets must be carefully curated to ensure they are diverse, representative, and free of harmful stereotypes. This involves identifying and mitigating biases in existing datasets and supplementing them with synthetic data that enhance representation.
Model Auditing and Fine-Tuning: Regular auditing of generative AI outputs is essential to detect and address emergent biases. Fine-tuning models using fairness-aware objectives or targeted datasets can help align outputs with desired ethical and societal values.
User Education and Transparency: Organizations deploying generative AI should communicate the limitations of these models, including their susceptibility to bias. Users should critically evaluate their outputs and provide feedback for improvement.
These measures reduce the risk of perpetuating bias and position organizations as leaders in ethical AI by building trust with customers and stakeholders. Generative AI, when responsibly managed, can become a tool for fostering inclusivity and creativity rather than reinforcing societal inequities.
The Path Forward
Bias in AI is not merely a technical challenge; it is a societal issue that requires holistic governance. Effective AI governance programs must prioritize fairness, transparency, and accountability at every stage of the AI lifecycle. By combining technical solutions with robust ethical and regulatory frameworks, organizations can mitigate harm, build trust, and unlock the full potential of AI.
Turning Bias into a Strategic Advantage
Rather than viewing bias solely as a flaw, enterprises should see it as a lens through which they can better understand how past values and priorities shaped outcomes. This understanding allows organizations to:
- Diagnose Root Causes of Inequities: Historical data can reveal where inequities originated and help frame discussions about necessary course corrections.
- Model the Implications of Change: AI can simulate how adjustments to values, policies, or priorities might play out, providing insights into the long-term impacts of strategic shifts.
Example: A company could use AI to analyze how adopting new hiring policies focused on diversity might reshape its workforce over time and correlate those changes with innovation, productivity, or cultural cohesion.
- Engage Stakeholders Proactively: Transparent communication about how an organization acknowledges and mitigates historical bias can build trust with stakeholders who increasingly demand alignment with ethical and social values.
The Role of AI in Understanding and Shaping Values
AI isn’t just a tool for automating decisions. It can be a mirror reflecting our priorities and a canvas for envisioning better futures. By leveraging AI, organizations can:
- Test Ethical Scenarios: Simulate different approaches to fairness or equity and predict their impacts, allowing decision-makers to choose policies that align with the organization’s goals and values.
- Quantify Trade-offs: Use AI to analyze the trade-offs between competing priorities (e.g., optimizing for profit vs. prioritizing equity) and make informed, balanced decisions.
- Enhance Accountability: Ensure that the ethical implications of AI systems are continually reviewed and aligned with evolving societal norms.
A Call to Action for CEOs and Boards
AI governance is not a "nice to have" but a critical business imperative. Reducing bias not only avoids regulatory fines but also enhances customer loyalty, opens access to new markets, and strengthens your brand reputation. To address the risk and societal impact of AI bias effectively, leaders should establish clear accountability such as by appointing AI Governance Officers and mandating annual bias audits as part of their enterprise risk management programs. An AI Governance Officer would be tasked with overseeing compliance, managing stakeholder expectations, and ensuring the ethical deployment of AI systems across the enterprise. These steps ensure leadership oversight, systemic evaluation, and sustained improvement in the fairness and equity of AI systems.
What Would AI Bias Audits Entail?
Bias audits are comprehensive evaluations aimed at detecting, understanding, and mitigating biases across the AI lifecycle. A robust AI bias audit program would include the following components:
- Dataset Evaluation:
- Objective: Assess training data for diversity, representation, and the presence of harmful patterns or omissions.
- Activities:
- Analyze demographic representation to ensure coverage across key groups.
- Identify and flag correlations that could lead to disparate impacts.
- Use tools like IBM AI Fairness 360 or Google's Dataset Search to scrutinize datasets for anomalies and gaps.
- Algorithmic Analysis:
- Objective: Evaluate models for inherent biases introduced during design and training.
- Activities:
- Test algorithms with fairness metrics like demographic parity, equalized odds, or disparate impact ratio. (Demographic parity ensures outcomes are equally distributed across demographic groups, regardless of their representation in the data.)
- Perform sensitivity analyses to understand how small changes in inputs impact outputs.
- Use explainability tools (e.g., SHAP, LIME) to uncover decision-making logic.
- Deployment Review:
- Objective: Ensure fairness in real-world conditions and assess user interaction biases.
- Activities:
- Monitor outputs in live environments for unexpected patterns or disparate impacts.
- Gather user feedback and conduct surveys to identify potential operational biases.
- Conduct periodic stress tests to evaluate the system under varied and extreme conditions.
- Stakeholder Reporting:
- Objective: Ensure transparency and accountability.
- Activities:
- Publish results of bias audits in board reports and sustainability disclosures.
- Engage external auditors or advisory panels for independent evaluation.
The Need for Ongoing Monitoring and Testing
AI systems are dynamic, with models evolving as they interact with new data and user behaviors. A one-time audit is insufficient to address the continuous risk of bias. Ongoing monitoring and testing are critical for maintaining fairness and trustworthiness:
- Real-Time Monitoring:
- Implement dashboards to track model performance and detect potential biases in real-time.
- Use anomaly detection tools to flag unusual patterns that may indicate emergent biases.
- Periodic Testing:
- Schedule quarterly or semi-annual tests to assess bias in updated models.
- Test for fairness across scenarios, including edge cases that might stress the system.
- Feedback Loops:
- Encourage stakeholders, including customers and employees, to report concerns.
- Use feedback to iteratively refine models and governance practices.
Conclusion
Bias in AI isn’t just a problem—it’s a responsibility. Historical data provides valuable insights into past realities, but enterprises must navigate the delicate balance between acknowledging those realities and building systems that reflect today’s values. Enterprises that embrace this challenge will help to mitigate risks and position themselves as leaders in ethical and responsible innovation.
AI should be used to identify and understand bias in other AI applications. Innovative leaders will also use AI to explore the implications of different ethical priorities and be architects of a fairer future where the benefits of AI accrue to many stakeholders. This requires a governance approach that combines technical rigor, ethical reflection, and strategic foresight - hallmarks of a market-leading AI governance program.
The journey to guard against bias is ongoing. It demands vigilance, continuous improvement, and collaboration across disciplines. Ultimately, the success of AI depends on our collective ability to create systems that serve all humanity equitably. Businesses, regulators, and technologists must join forces to champion responsible AI governance. Let’s make bias-free AI a foundation of trustworthy innovation.
Featured in: AI / Artificial Intelligence