Why Purpose-Built Solutions Are Essential for Governing AI
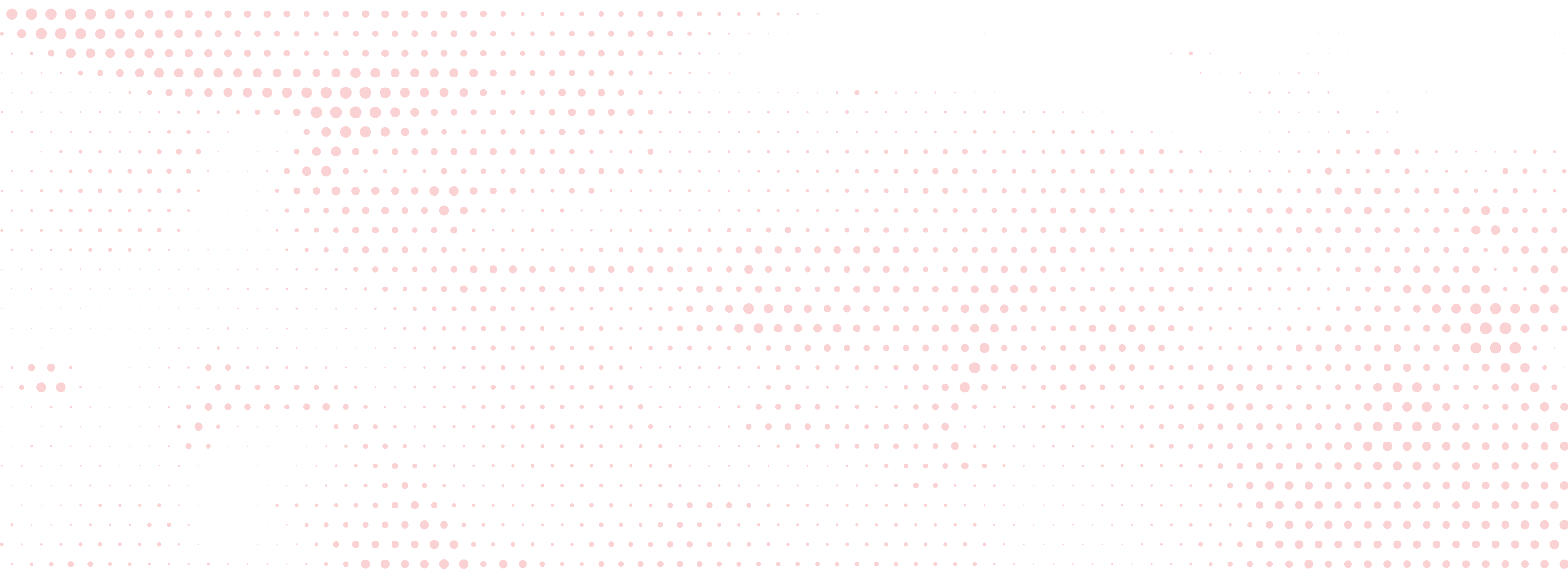
This blog discusses why purpose-built AI governance solutions are essential for enterprises to effectively manage the unique risks, compliance challenges, and technical complexities inherent in artificial intelligence systems.
As artificial intelligence (AI) continues to reshape industries, enterprises face unprecedented challenges in governing its use, managing its risks, and ensuring compliance with a growing web of global regulations. Traditional IT solutions, including but not limited to governance, risk management, and compliance (GRC) tools, built for static processes and conventional risks, cannot adequately address AI's new, dynamic, and multifaceted challenges. Purpose-built AI solutions are not optional for governing AI - they are indispensable.
In this 12th blog of the AI Governance series, we will explore why purpose-built solutions are essential, identify the shortcomings of legacy tools, and outline key features enterprises must prioritize to govern AI effectively.
The Case for Purpose-Built AI Solutions
AI introduces unique complexities and risks that differ fundamentally from those faced by traditional IT systems. From ethical concerns like algorithmic bias to technical challenges such as explainability and adversarial attacks, AI requires governance approaches that can address its specific demands.
Key Reasons Why Traditional Tools Fall Short:
- AI-Specific Risks: Traditional tools are ill-equipped to handle risks such as:
- Bias and Discrimination: Identifying and mitigating bias requires specialized algorithms and datasets.
- Explainability: Understanding AI decisions necessitates tools capable of interpreting complex models like neural networks.
- Security Vulnerabilities: Defending against adversarial attacks is beyond the scope of many legacy solutions.
- Regulatory Complexity: AI-specific laws, such as the EU's AI Act and emerging frameworks in the U.S. and Asia, demand specialized compliance capabilities. Legacy systems often lack the adaptability to address these evolving requirements.
- Lifecycle Demands: AI models require continuous monitoring, retraining, and updating - tasks that cannot be managed effectively with static workflows or manual oversight.
- Technical Complexity: The intricate nature of AI models, data dependencies, and evolving architectures demand tools with deep technical integration and insight, that leverage AI to manage, test, audit, and analyze AI systems.
- Scalability Challenges: As enterprises scale their AI deployments, traditional tools struggle to provide the oversight, automation, and real-time responsiveness needed to manage portfolios of AI models.
What Enterprises Should Look for in Purpose-Built AI Solutions
To effectively govern AI, enterprises need solutions designed specifically for the unique challenges AI presents. These solutions should provide comprehensive capabilities across several key dimensions.
- System of Record
- A centralized repository for all AI models, datasets, and processes.
- Full traceability for development, deployment, and monitoring stages.
- Documented compliance with internal and external standards.
- Portfolio of Testing Tools
- Advanced capabilities for detecting bias, ensuring fairness, and validating explainability.
- Security testing to identify and mitigate vulnerabilities to adversarial attacks.
- Tools for validating data quality and lineage to ensure trustworthy AI outputs.
- Regulatory Compliance Features
- Built-in frameworks for adhering to global AI regulations, with updates for new laws.
- Reporting templates for audits and regulatory submissions.
- Continuous tracking of compliance metrics across the AI lifecycle.
- Lifecycle Management
- Monitoring and alerting for model performance, including drift detection.
- Automated retraining workflows to maintain accuracy and relevance.
- Real-time updates to policies and processes as regulatory landscapes evolve.
- Integration with Enterprise Ecosystems
- APIs for seamless connections with existing tools, such as data warehouses and business intelligence platforms.
- Support for multi-cloud and hybrid environments, enabling consistent governance across diverse infrastructures.
Advanced Features for Modern AI Governance
A robust purpose-built platform should go beyond the basics, providing tools that enable enterprises to manage their AI ecosystems with precision and agility:
- Workflow and Collaboration Tools:
- Enable cross-functional collaboration among data scientists, engineers, compliance officers, and business leaders.
- Support approval processes and development tracking.
- Automated Risk Assessment:
- Use AI to predict risks and score potential vulnerabilities before deployment.
- Provide actionable recommendations to mitigate risks.
- Incident Management:
- Track and resolve AI-related incidents, ensuring issues are documented and addressed.
- Facilitate root cause analysis and implement lessons learned.
- Training and Education:
- Provide role-specific training for AI governance best practices.
- Enhance AI literacy across all levels of the organization.
- Data Governance Tools:
- Manage data quality, lineage, and privacy with AI-enhanced tools.
- Ensure datasets meet ethical and legal standards for training and operations.
- Build and implement organizational-specific ontology to organize raw data,
- Transparency and Accountability:
- Embed explainability into the development process.
- Provide stakeholders with clear, understandable insights into AI decisions.
Addressing Common Objections to Purpose-Built Solutions
While the need for specialized AI governance tools is clear, organizations often raise several concerns about adopting purpose-built solutions. Let's address these directly:
- Cost Considerations
- Objection: Purpose-built solutions represent a significant investment
- Reality: The cost of inadequate governance—including regulatory fines, reputational damage, and AI failures—typically far exceeds the investment in proper tools
- ROI: Purpose-built solutions often reduce total cost of ownership through automation, efficiency gains, and risk reduction
- Integration Challenges
- Objection: New solutions might not integrate well with existing systems
- Reality: Modern purpose-built platforms are designed with robust APIs and integration capabilities
- Benefit: Proper integration enhances overall governance effectiveness by creating a unified view across systems
- Resource Requirements
- Objection: Implementation and maintenance demand significant resources
- Reality: Purpose-built solutions often reduce resource requirements through automation and streamlined workflows
- Outcome: Teams can focus on strategic governance rather than manual oversight
- Vendor Lock-in Concerns
- Objection: Organizations fear becoming dependent on a single vendor
- Reality: The best solutions support open standards and provide data portability
- Strategy: Choose vendors committed to interoperability and standard formats
Enhanced Validation and Testing Requirements
Purpose-built solutions must provide comprehensive validation capabilities across the AI lifecycle:
- Model Validation Framework
- Automated testing suites for model performance
- Validation against defined benchmarks
- Cross-validation capabilities
- Performance degradation detection
- Data Validation Tools
- Data quality assessment
- Distribution analysis
- Drift detection
- Anomaly identification
- Documentation and Audit Trail
- Automated documentation generation
- Version control for models and data
- Change tracking and approval workflows
- Comprehensive audit logs
- Testing Frameworks
- Unit testing for model components
- Integration testing for AI systems
- Stress testing under various conditions
- A/B testing capabilities
Regulatory Compliance and Documentation
New AI regulations increasingly demand capabilities that only purpose-built solutions can provide:
- Regulatory Requirements
- EU AI Act compliance features
- DOJ guidance alignment
- Model risk management controls
- Privacy regulation compliance (GDPR, CCPA)
- Documentation Requirements
- Model development documentation
- Training data validation
- Decision audit trails
- Impact assessments
- Reporting Capabilities
- Automated compliance reporting
- Risk assessment documentation
- Incident response tracking
- Performance monitoring reports
Implementation Considerations
To successfully adopt purpose-built solutions, organizations should:
- Assess Current State
- Evaluate existing governance tools
- Identify gaps and pain points
- Define specific requirements
- Plan the Transition
- Develop a phased implementation approach
- Define success metrics
- Create training and change management plans
- Ensure Stakeholder Alignment
- Secure executive sponsorship
- Engage key stakeholders
- Develop communication strategy
Building a Future-Proof AI Governance Strategy
The decision to invest in purpose-built AI solutions is not just about addressing today’s challenges; it is a strategic imperative to prepare for the future. As AI continues to evolve and regulations tighten, enterprises that proactively implement these solutions will position themselves as leaders in trust, innovation, and sustainability.
By adopting platforms that provide a comprehensive system of record, advanced testing tools, and seamless lifecycle management, organizations can unlock AI's transformative potential while safeguarding against its risks. Purpose-built solutions ensure enterprises remain agile and resilient in the face of rapid change, ultimately enabling them to thrive in the era of AI.
Final Thoughts
Managing AI with repurposed legacy tools will not succeed. Purpose-built AI governance solutions are not just an option but a necessity. Enterprises that embrace this reality will be better equipped to navigate the complexities of AI, maintain compliance, and drive innovation responsibly.
As the AI governance landscape continues to evolve, organizations must act decisively to adopt tools that meet the unique demands of this transformative technology. With the right solutions, enterprises can build a governance framework that mitigates risks and maximizes the opportunities AI offers. This is how businesses will transition from reactive compliance to proactive leadership in the age of AI.
Featured in: AI / Artificial Intelligence